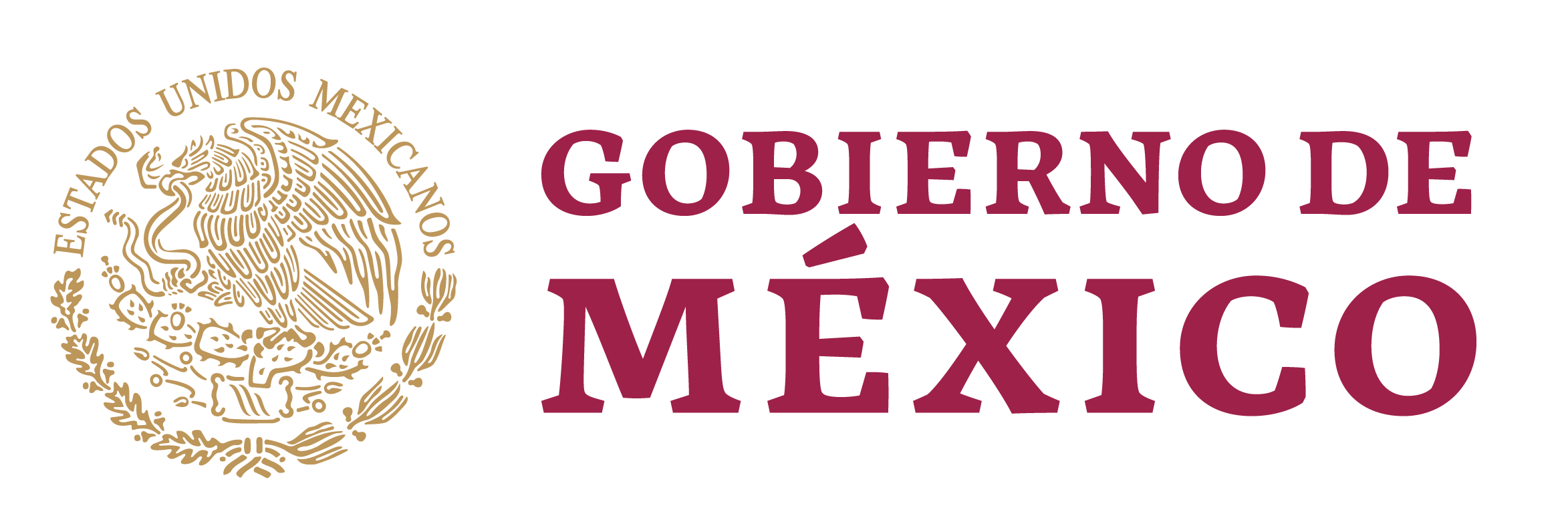
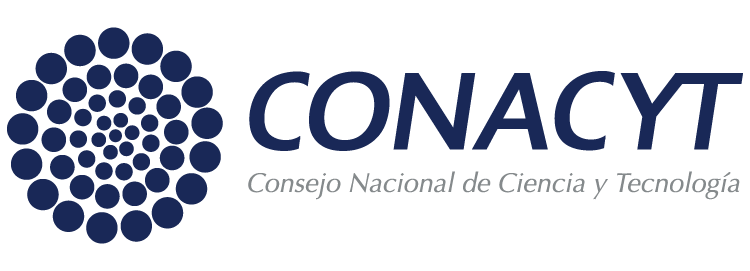
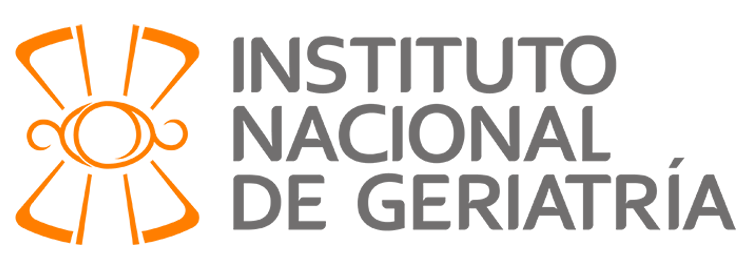
Por favor, use este identificador para citar o enlazar este ítem:
http://repositorio.inger.gob.mx/jspui/handle/20.500.12100/17308
Registro completo de metadatos
Campo DC | Valor | Lengua/Idioma |
---|---|---|
dc.rights.license | http://creativecommons.org/licenses/by/4.0 | es_MX |
dc.creator | OMAR YAXMEHEN BELLO CHAVOLLA | es_MX |
dc.creator | JESSICA PAOLA BAHENA LOPEZ | es_MX |
dc.creator | ARSENIO VARGAS VAZQUEZ | es_MX |
dc.creator | Neftali Eduardo Antonio Villa | es_MX |
dc.creator | Alejandro Márquez Salinas | es_MX |
dc.creator | Carlos Alberto Fermín Martínez | es_MX |
dc.creator | MARIA ROSALBA ROJAS MARTINEZ | es_MX |
dc.creator | ROOPA PRAVIN MEHTA | es_MX |
dc.creator | IVETTE CRUZ BAUTISTA | es_MX |
dc.creator | MIGUEL SERGIO HERNANDEZ JIMENEZ | es_MX |
dc.creator | ANA CRISTINA GARCIA ULLOA | es_MX |
dc.creator | PALOMA ALMEDA VALDES | es_MX |
dc.creator | CARLOS ALBERTO AGUILAR SALINAS | es_MX |
dc.date | 2020 | - |
dc.date.accessioned | 2021-11-17T19:22:59Z | - |
dc.date.available | 2021-11-17T19:22:59Z | - |
dc.identifier.uri | http://repositorio.inger.gob.mx/jspui/handle/20.500.12100/17308 | - |
dc.description | Introduction Previous reports in European populations demonstrated the existence of five data-driven adult-onset diabetes subgroups. Here, we use self-normalizing neural networks (SNNN) to improve reproducibility of these data-driven diabetes subgroups in Mexican cohorts to extend its application to more diverse settings. Research design and methods We trained SNNN and compared it with k-means clustering to classify diabetes subgroups in a multiethnic and representative population-based National Health and Nutrition Examination Survey (NHANES) datasets with all available measures (training sample: NHANES-III, n=1132; validation sample: NHANES 1999–2006, n=626). SNNN models were then applied to four Mexican cohorts (SIGMA-UIEM, n=1521; Metabolic Syndrome cohort, n=6144; ENSANUT 2016, n=614 and CAIPaDi, n=1608) to characterize diabetes subgroups in Mexicans according to treatment response, risk for chronic complications and risk factors for the incidence of each subgroup. Results SNNN yielded four reproducible clinical profiles (obesity related, insulin deficient, insulin resistant, age related) in NHANES and Mexican cohorts even without C-peptide measurements. We observed in a population-based survey a high prevalence of the insulin-deficient form (41.25%, 95% CI 41.02% to 41.48%), followed by obesity-related (33.60%, 95% CI 33.40% to 33.79%), age-related (14.72%, 95% CI 14.63% to 14.82%) and severe insulin-resistant groups. A significant association was found between the SLC16A11 diabetes risk variant and the obesity-related subgroup (OR 1.42, 95% CI 1.10 to 1.83, p=0.008). Among incident cases, we observed a greater incidence of mild obesity-related diabetes (n=149, 45.0%). In a diabetes outpatient clinic cohort, we observed increased 1-year risk (HR 1.59, 95% CI 1.01 to 2.51) and 2-year risk (HR 1.94, 95% CI 1.13 to 3.31) for incident retinopathy in the insulin-deficient group and decreased 2-year diabetic retinopathy risk for the obesity-related subgroup (HR 0.49, 95% CI 0.27 to 0.89). Conclusions Diabetes subgroup phenotypes are reproducible using SNNN; our algorithm is available as web-based tool. Application of these models allowed for better characterization of diabetes subgroups and risk factors in Mexicans that could have clinical applications. | es_MX |
dc.format | Adobe PDF | es_MX |
dc.language | eng | es_MX |
dc.publisher | BMJ Publishing Group | es_MX |
dc.relation | https://drc.bmj.com/content/8/1/e001550.long | es_MX |
dc.relation.requires | Si | es_MX |
dc.rights | Acceso Abierto | es_MX |
dc.source | BMJ Open Diabetes Research and Care (2052-4897) Vol. 8 (2020) | es_MX |
dc.subject | MEDICINA Y CIENCIAS DE LA SALUD | es_MX |
dc.subject | Ciencias médicas | es_MX |
dc.subject | Ciencias clínicas | es_MX |
dc.subject | Geriatría | es_MX |
dc.subject | Diabetes | es_MX |
dc.subject | Diabetes mellitus | es_MX |
dc.subject | Obesidad | es_MX |
dc.subject | Obesity | es_MX |
dc.subject | Encuesta de Salud Nacional y Examen de Nutrición | es_MX |
dc.subject | National Health and Nutrition Examination Survey | es_MX |
dc.subject | México | es_MX |
dc.subject | Mexico | es_MX |
dc.title | Clinical characterization of data-driven diabetes subgroups in Mexicans using a reproducible machine learning approach | es_MX |
dc.type | Artículo | es_MX |
dc.audience | Researchers | es_MX |
dc.creator.id | BECO920925HDFLHM05 | es_MX |
dc.creator.id | BALJ930827MDFHPS02 | es_MX |
dc.creator.id | VAVA940920HGRRZR01 | es_MX |
dc.creator.id | CA1343917 | es_MX |
dc.creator.id | CA1343931 | es_MX |
dc.creator.id | CA1343932 | es_MX |
dc.creator.id | ROMR600708MPLJRS08 | es_MX |
dc.creator.id | MEXR720429MNEHXP03 | es_MX |
dc.creator.id | CUBI740301MDFRTV06 | es_MX |
dc.creator.id | HEJM631007HDFRMG01 | es_MX |
dc.creator.id | GAUA801214MDFRLN04 | es_MX |
dc.creator.id | AEVP760218MDFLLL01 | es_MX |
dc.creator.id | AUSC611116HDFGLR00 | es_MX |
dc.creator.nameIdentifier | curp | es_MX |
dc.creator.nameIdentifier | curp | es_MX |
dc.creator.nameIdentifier | curp | es_MX |
dc.creator.nameIdentifier | ca | es_MX |
dc.creator.nameIdentifier | ca | es_MX |
dc.creator.nameIdentifier | ca | es_MX |
dc.creator.nameIdentifier | curp | es_MX |
dc.creator.nameIdentifier | curp | es_MX |
dc.creator.nameIdentifier | curp | es_MX |
dc.creator.nameIdentifier | curp | es_MX |
dc.creator.nameIdentifier | curp | es_MX |
dc.creator.nameIdentifier | curp | es_MX |
dc.creator.nameIdentifier | curp | es_MX |
Aparece en las colecciones: | 1. Artículos |
Ficheros en este ítem:
Fichero | Descripción | Tamaño | Formato | |
---|---|---|---|---|
BMJ Open Diabetes Research and Care (2052-4897) Vol. 8 (2020).pdf | 1.03 MB | Adobe PDF | Visualizar/Abrir |